
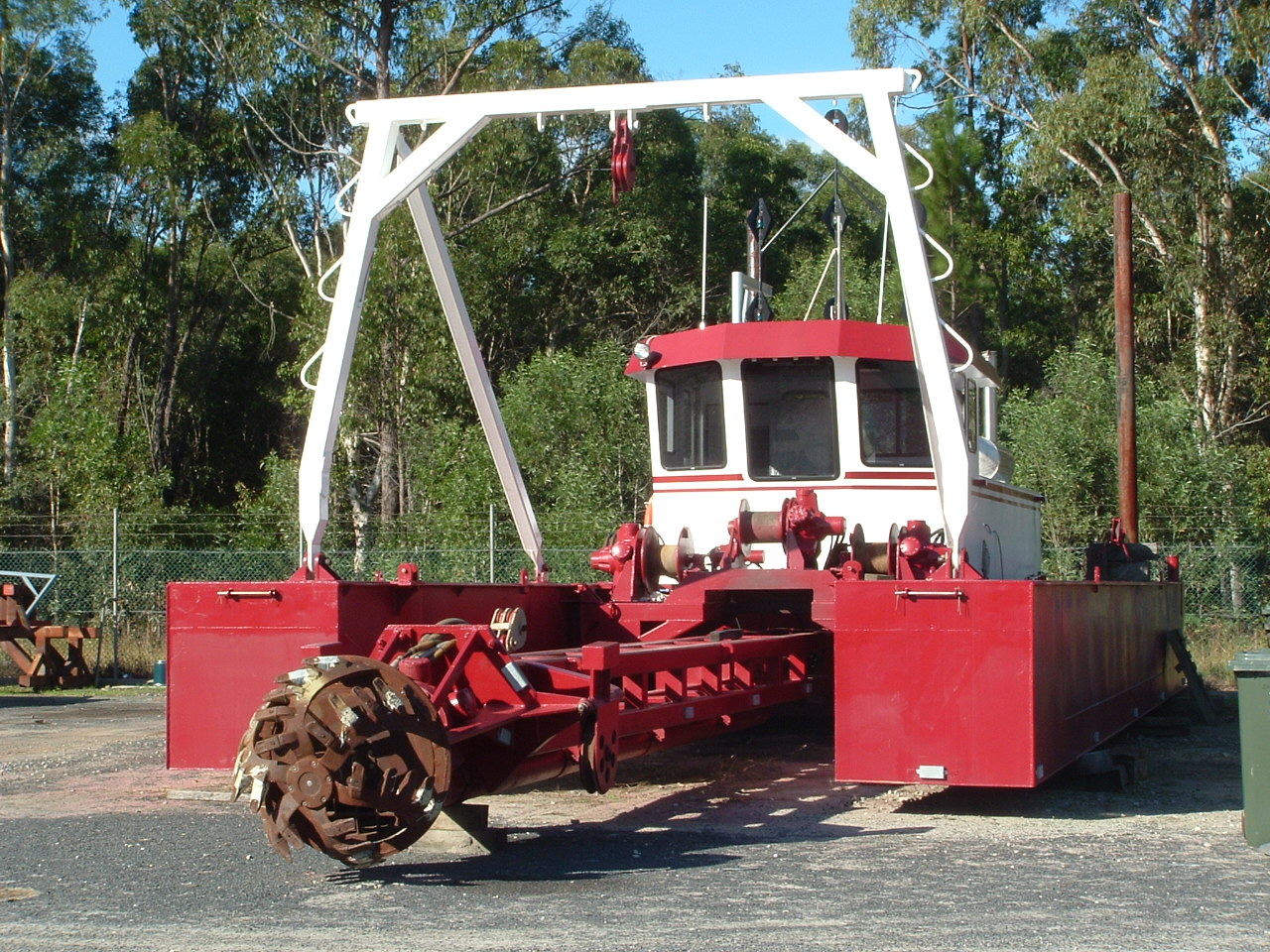
Another instance that is closely associated with this phenomenon is the recent trend of multicentre studies. Ioannidis (2005) explains that ‘negative’ research is useful and, in fact, it is a misnomer to classify it as negative.

Therefore, it should be understood that statistical inference only tells us about the range of the truth within the data that has been observed. Part of the reason for this is also because the difference between inferential and descriptive use of statistics is often blurred, and could be mistaken by novice epidemiologists. A significant part of the statistical estimate is based on the assumption that the correct statistical model is estimated. The predominant reason for this practice is the widespread notion among academics that “statistically significant data is noteworthy, and one that is not statistically significant is not”. We may use these term interchangeably in the discussion below. Data dredging is recognized by several names such as ‘fishing trip’, ‘data snooping’, ‘p-hacking’ and so on. This may lead to an exponential increase in the risk of inclusion of large quantities of false positive results, thereby corrupting the data that was meant to be originally reported.

